We live in a day and age where information can be mathematically determined with the aid of statistics. However, the study of statistics, as it seems, isn’t simply that of facts and numbers.
Statistical inference consists of the use of statistics to create decisions concerning the parameters of a population, based on random sampling. The implementation of statistical inference involves hypothesis testing and talks about how this procedure is employed by statisticians to just accept or reject the assumption of a population parameter. U
Key Takeaways
- A paired t-test is a statistical method used to compare the means of two related samples, such as measurements taken from the same individuals at different times or under different conditions.
- An unpaired t-test, also known as an independent samples t-test, compares the means of two unrelated samples, such as measurements from two groups of individuals.
- The choice between a paired and unpaired t-test depends on the nature of the data and the research question, with paired t-tests used for related samples and unpaired t-tests for independent samples.
Paired T-Test vs Unpaired T-Test
A paired t-test is a statistical test used to compare the means of two related samples; in this, samples are paired or matched in some way. The paired t-test is used when there is a natural pairing between two samples. An unpaired t-test is a statistical test used to compare the means of two independent samples. The unpaired t-test is used when there is no natural pairing between the two samples.
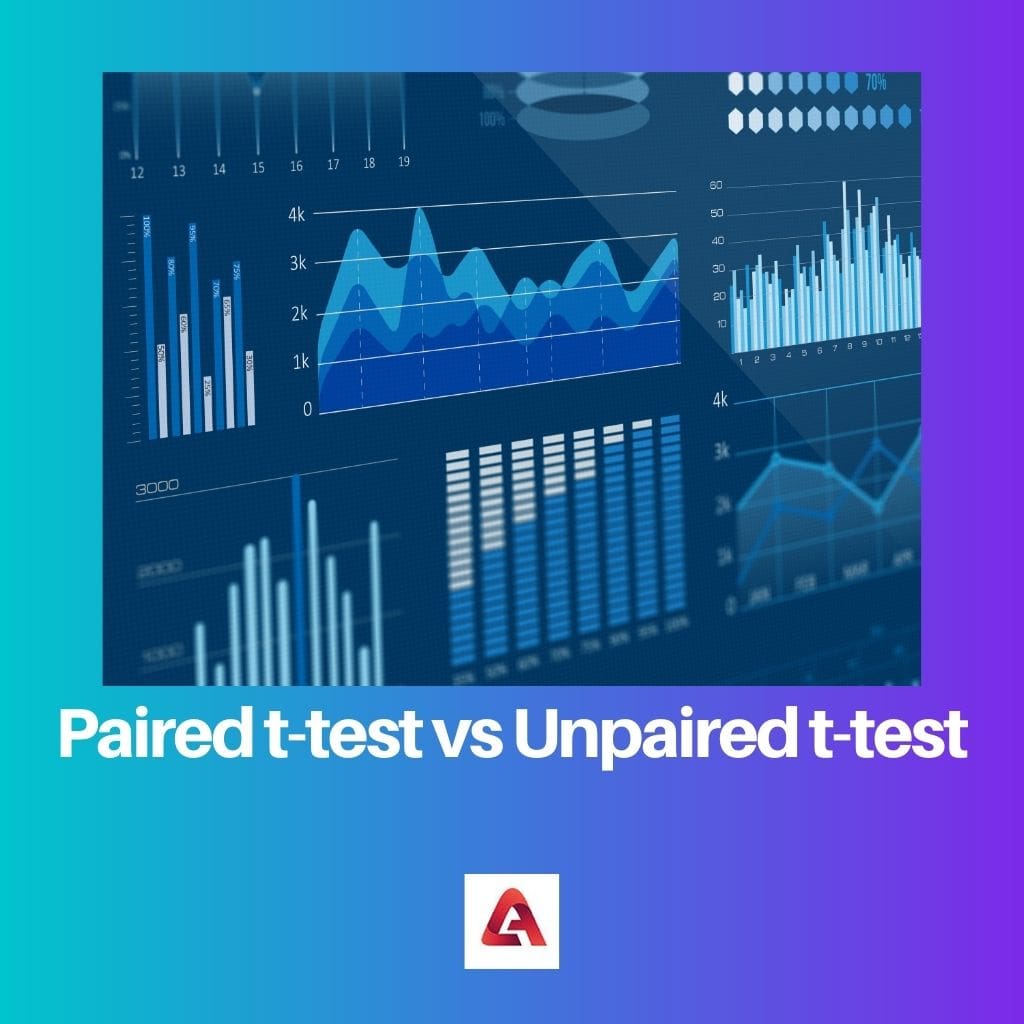
Comparison Table
Parameter of comparison | Paired T-Test | Unpaired T-Test |
---|---|---|
Meaning | Paired T-Test, also known as repeated samples T-Test, determines the distinction between the two means of the same subject. | Unpaired T-Tests, also known as independent T-Tests or student’s T-Test, is determining the two means groups of different/unrelated subjects. |
Homogeneity of variances | Under Paired T-Test the variance of the two mean groups are not equal. | Under Unpaired T-Test the variance of the two mean groups are equal. |
Effects/impacts | Paired T-tests deal with very minor errors since the test is done only between two similar groups. | Unpaired T-Tests have slightly more errors in comparison with paired T-Tests since the experimenter would be affected by variations between two different subjects. |
Outcome | Paired T-Tests need not collect massive amounts of sample data for comparison this successively saves money and time. | Since Unpaired T-Tests have to compare the means of two independent subjects this lands up being a slightly more costlier and time-consuming process. |
What is Paired T-Test?
A Paired T-Test, additionally referred to as correlated pair t-test/paired sample t-test/dependent t-test, is a statistical procedure that runs a test on dependent variables. A paired test is done on similar subjects before the allocation of data and two tests are done before and after a treatment.
Hypothesis:
The two hypotheses under paired t-test.
- The null hypothesis (H0): no significant difference between specified populations, H0: ÎĽ1 = ÎĽ2
- The alternative hypothesis (H1): there is a statistically significant difference between the two population means caused by rejecting the null hypothesis. H1: μ 1 ≠μ2
Assumptions:
The paired sample t-test makes the subsequent assumptions:
- The differences between the similar pairs follow a normal probability distribution.
- The observations ought to be sampled independently and identically distributed.
- A Paired t-test is measured on a gradual level with the help of ratios or intervals. Since T-Tests are based on a normal distribution, the data needs to be continuous and not discrete
- The independent variables should comprise of two dependent/similar groups.
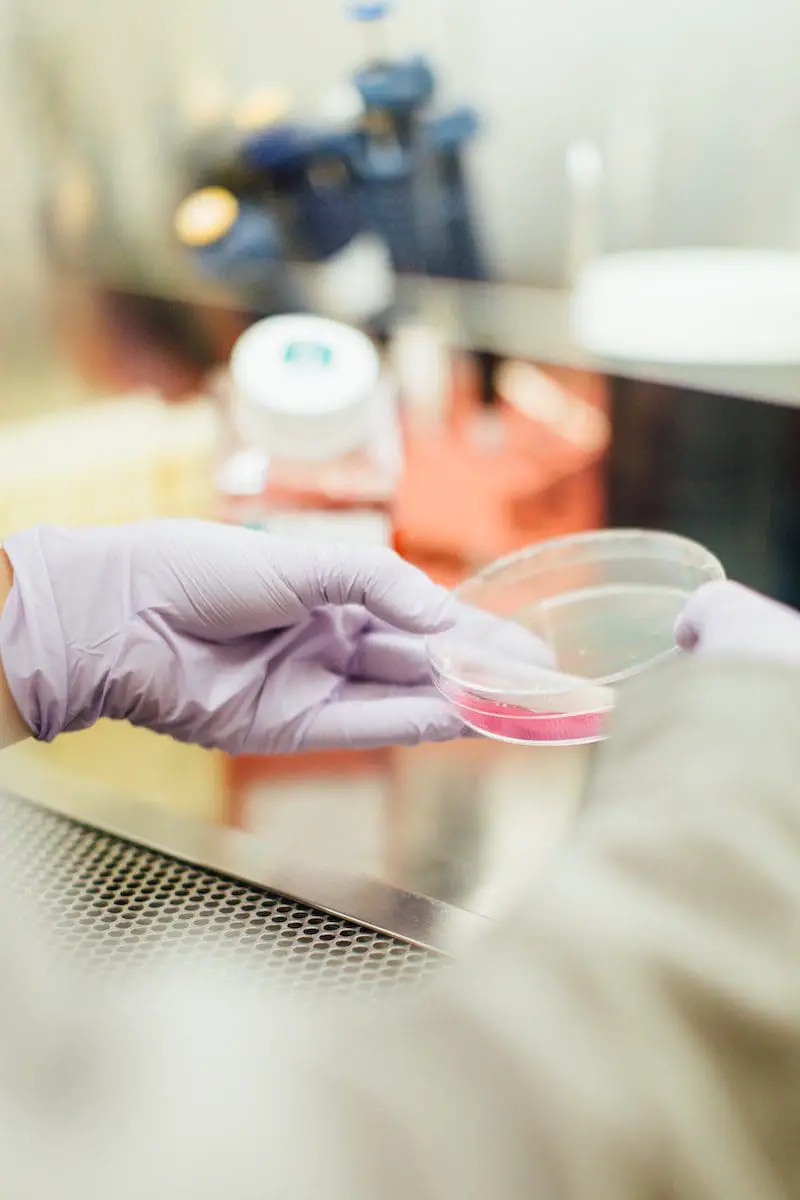
What is Unpaired T-Test?
An unpaired t-test, also known as an independent sample t-test/two-sample t-test, is a statistical method that determines whether or not there is a significant distinction between the means of two unrelated independent groups. For example: when you want to compare the average sleep cycle of individuals grouped by gender: male and female groups.
Hypothesis for the independent t-test:
The null hypothesis for the independent t-test is that the population means from the two different groups are equal:
H0: ÎĽ1= ÎĽ2
Alternative hypothesis is accepted once the null hypothesis is rejected, which means that the population means are not equal
H1: μ1 ≠μ2
To reject or accept the null hypothesis, a significance level is critical. This particular value is 0.05.
Assumptions:
- The first assumption concerns the scale of measurement- the data collected ought to follow a continuous or ordinal scale.
- The data should be collected from a randomly selected portion of the total population.
- The data should result in a normal, bell-shaped distribution curve. The significance level can be specified when a normal distribution is assumed.
- A massive sample size ought to be used.
- The variance and standard deviations should be equal for the dependent variables.
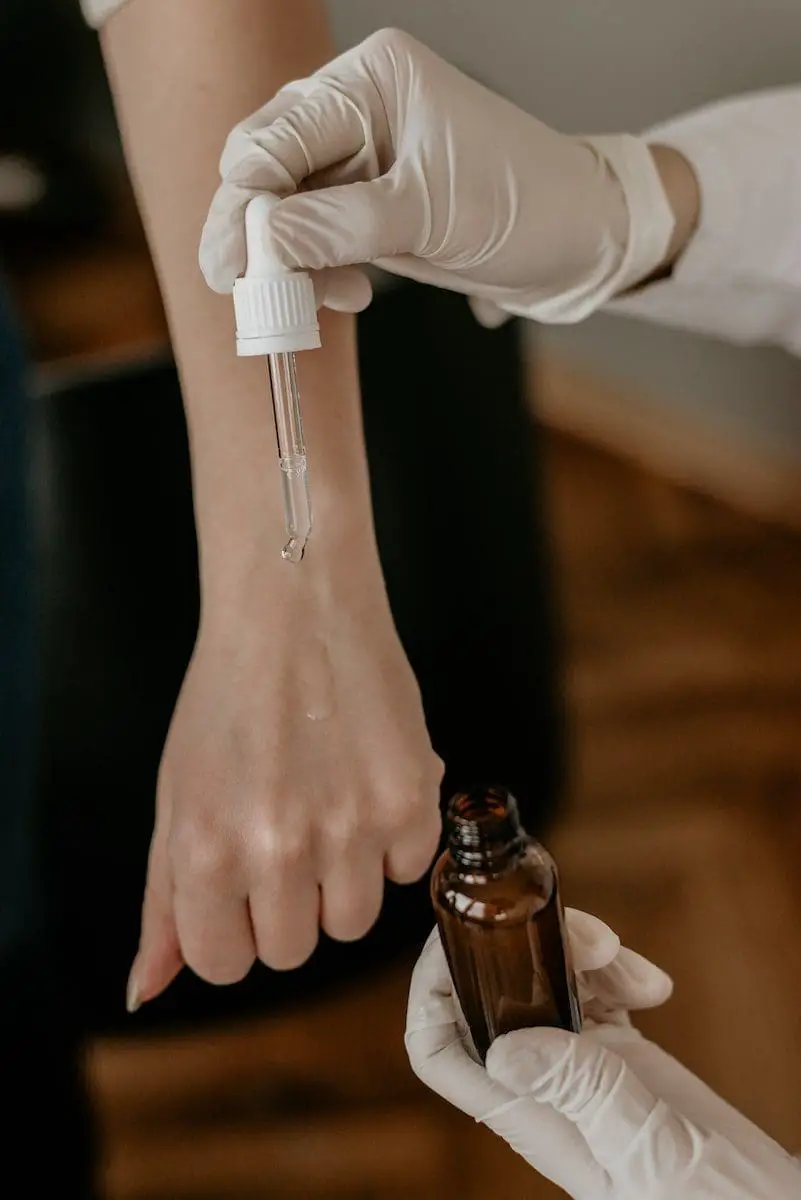
Main Differences Between Paired T-Test and Unpaired T-Test
- Paired T-Tests means comparing the difference between the two mean groups of dependent subjects. For example: the IQ of 5 students before and after training.
- The variance of Paired T-Tests is said to be equal. Since the variance is equal standard deviation also is equal for the two mean groups.
- Paired T-Tests has less random errors since Paired T-Tests mainly deal with finding the variations between two mean groups of similar subjects the experimenter need not focus on the individual differences.
- Paired T-Tests saves heaps of time and money for the experimenter as he need not find large amounts of sample data to calculate the two similar mean groups. Unpaired T-Tests are slightly costlier and time-consuming process as the experimenter would have to find a lot of data to analyze the two independent mean groups.