In statistics, there are several concepts that help us to reach a particular result. Statistical data can vary from content to content and quantity to quantity.
Statistics is a type of branch that helps us to acquire a rough idea regarding an ongoing event. It helps us to predict the results and thereby make decisions regarding the same.
Statistical analysis is done on the basis of various data that is collected during a certain event or after it. However, various types of data are analyzed by using various types of concepts.
Two of such concepts are 1. OLS or ordinary least squares and 2. MLE or maximum likelihood estimation.
Key Takeaways
- Ordinary Least Squares (OLS) is a statistical method for estimating linear regression models by minimizing the sum of squared errors.
- Maximum Likelihood Estimation (MLE) is a statistical technique that estimates parameters by maximizing the likelihood function.
- OLS is specific to linear regression, whereas MLE can be applied to various statistical models.
OLS vs MLE
OLS estimates the parameters that minimize the sum of the squared residuals, while MLE estimates the parameters that maximize the likelihood of the observed data. OLS is a simpler and more intuitive method, while MLE can handle more complex models and be more efficient in small samples.
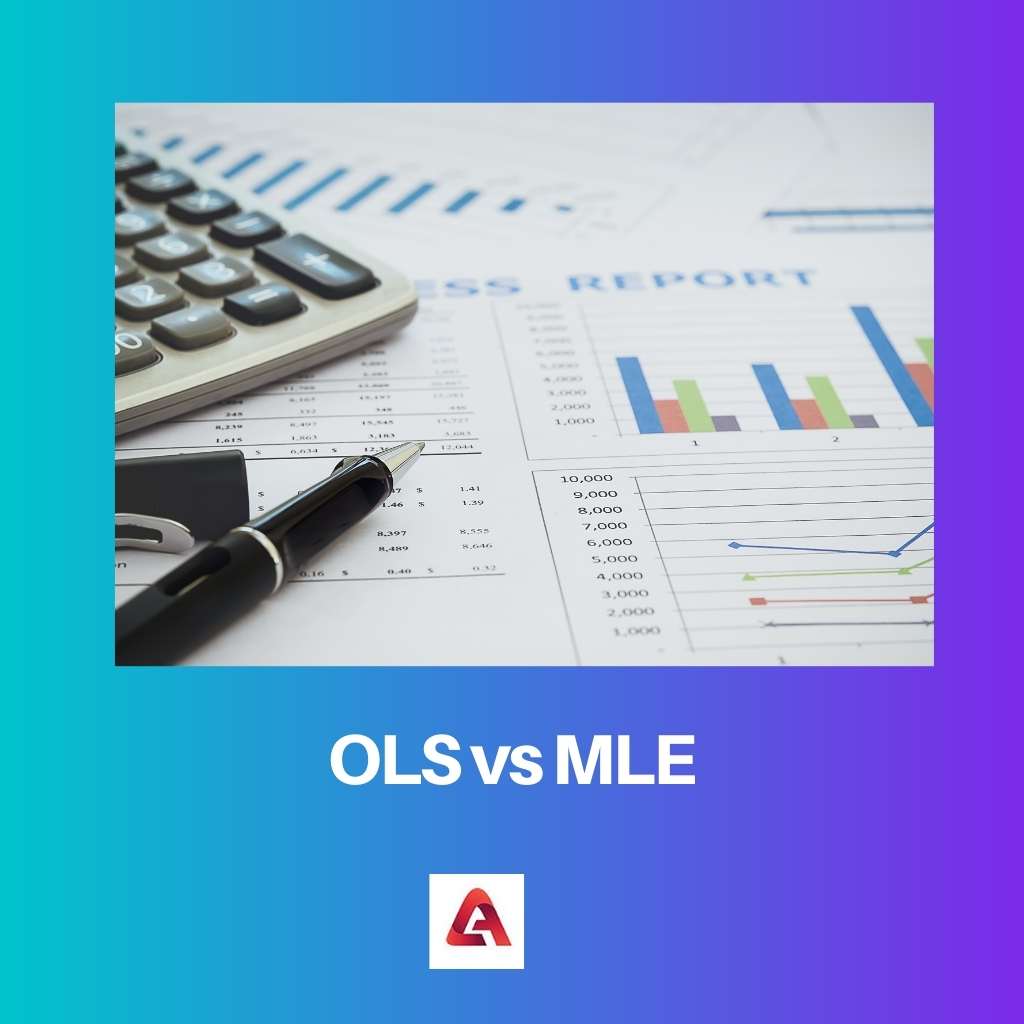
The method used to calculate and estimate the unknown parameters present in a certain linear regression model is known as ordinary least squares (OLS). It is a method in which the number of errors is equally distributed.
It is one of the most consistent techniques when the regressors in the model originate externally.
The method in statistics that is used to estimate several parameters when the probability distribution is assumed of the observed statistical data is known as the maximum likelihood estimation (MLE).
The maximum likelihood estimation is the point in the parameter space that maximizes the likelihood function.
Comparison Table
Parameters Of Comparison | OLS | MLE |
---|---|---|
Fullforms | Ordinary least squares. | Maximum likelihood estimation. |
Also known as | Linear least squares | No other name |
Used for | The ordinary least squares method is used for the determination of various unknown parameters that are present in a linear regression model. | The maximum likelihood estimation is the method that is used for 1. Parameter estimation 2. Fitting a statistical model to the statistical data. |
Discovered by | Adrien Marie Legendre | The concept was collectively derived with the help of the contributions done by Gauss, Hagen and Edgeworth. |
Drawbacks | It is not available and applicable to statistical data that is censored. It cannot be applied to data that has extremely big values or extremely small values. There are comparatively fewer optimality properties in this concept. | During the calculation of statistical data that has extremely smaller values the maximum likelihood estimation method can be quite biased, In some cases the one may need to specifically solve the likelihood equations, Sometimes the estimation of the numerical values can be non-trivial. |
What is OLS?
The method used to calculate and estimate the unknown parameters present in a certain linear regression model is known as ordinary least squares (OLS). The discovery of this concept in the world of statistics was made by Adrien Marie Legendre.
The frameworks in which the ordinary least squares is applicable may vary.
One must have to select an appropriate framework where the ordinary least squares can be cast in a particular linear regression model to find out the unknown parameters located in the same.
One of the aspects of this concept that is differential is whether to treat the regressors as random variables or as constants with predefined values.
If the regressors are treated as random variables, then the study can be more innate, and the variables can be samples together for a collective observational study. This leads to some comparatively more accurate results.
However, if the regressors are treated as constants with predefined values, then the study is considered comparatively more like an experiment.
There exist another classical linear regression model in which the emphasis is put on the sample data that is finite. This leads to a conclusion that the values in the data are limited and fixed, and the estimation of the data is done on the basis of the fixed data.
Further inference of the statistical is also calculated in a comparatively easier method.
What is MLE?
The method in statistics that is used to estimate several parameters when the probability distribution is assumed of the observed statistical data is known as the maximum likelihood estimation (MLE).
It has comparatively more optimal properties than many other concepts that are used to calculate the unknown parameters in various statistical models.
The initial estimation is done on the basis of the basic likelihood function of the statistical sample data.
Roughly prediction of the data is made like the set of data, and its likelihood is also the probability of obtaining a similar set of data for the given probability statistical model.
The entire rough prediction of the set of data consists of various unknown parameters that are located across the probability model. These values or these unknown parameters maximize the likelihood of the set of data.
These values are known as the maximum likelihood estimates. There exist several likelihood functions that are also useful for the distributions that are used commonly in reliability analysis.
There were exist censored models under which the censored data in reliability analysis is calculated, and the concept of maximum likelihood estimation can be used for doing the same.
Various parameters can be estimated by using this concept as it gives a comparatively more consistent approach towards it.
Several hypothesis sets can be generated for the parameters in the data by using this concept. It approximately contains both normal distributions as well as sample variances.
Main Differences Between OLS and MLE
- The OLS method is the ordinary least squares method. On the other hand, the MLE method is the maximum likelihood estimation.
- The ordinary linear squares method is also known as the linear least-squares method. On the other hand, the maximum likelihood method has no other name by which it is known.
- The ordinary least squares method has comparatively fewer optimal properties. On the other hand, the maximum likelihood estimation has comparatively more optimal properties.
- The ordinary least squares method can not be used for censored data. On the other hand, the maximum likelihood estimation method can be used for censored data.
- The ordinary least squares method is used for the determination of various unknown parameters that are present in a linear regression model. On the other hand, the maximum likelihood estimation is the method that is used for 1. Parameter estimation 2. Fitting a statistical model to the statistical data.
- https://methods.sagepub.com/base/download/BookChapter/the-multivariate-social-scientist/d49.xml
- https://rss.onlinelibrary.wiley.com/doi/abs/10.1111/j.2517-6161.1961.tb00430.x
