To understand the difference between PDF and PMF, it is essential to understand what Random variables are. A random variable is a variable whose value is not known to the task; in other words, the value depends on the result of the experiment.
For instance, while flipping a coin, the value, i.e. heads or tails, depends upon the outcome.
Key Takeaways
- PDF (Probability Density Function) is a statistical function used to describe the probabilities of continuous random variables within a given range.
- PMF (Probability Mass Function) is a statistical function that describes the probabilities of discrete random variables, assigning a probability to each possible outcome.
- PDF and PMF represent the probability distributions of random variables, but they differ in their application, with PDF used for continuous variables and PMF for discrete variables.
PDF vs PMF
PDF, also known as the probability density function, is a mathematical function that is used when there is a solution to be found within a range of continuous random variables. PMF, also known as probability mass function is a function that used discrete random variables to find a solution.

PDF and PMF are related to physics, statistics, calculus, or higher math. PDF (Probability Density Function) is the likelihood of the random variable in the range of discrete values.
On the other hand, PMF (Probability Mass Function) is the likelihood of the random variable in the range of continuous values.
Comparison Table
Parameter of Comparison | PMF | |
---|---|---|
Full form | Probability Density Function | Probability Mass Function |
Use | PDF is used when there is a need to find a solution in a range of continuous random variables. | PMF is used when finding a solution in a range of discrete random variables is needed. |
Random Variables | PDF uses continuous random variables. | PMF uses discrete random variables. |
Formula | F(x)= P(a < x 0 | p(x)= P(X=x) |
Solution | The solution falls in the radius range of continuous random variables | The Solutions fall in the radius between numbers of discrete random variables |
What is PDF?
The Probability Density Function (PDF) depicts probability functions in terms of continuous random variable values between a precise range of values.
It is also known as a probability distribution function or a probability function. It is denoted by f(x).
The PDF is essentially a variable density over a given range. It is positive/non-negative at any given point in the graph, and the full PDF always equals one.
In a case where the probability of X on some given value x (continuous random variable) is always 0. P(X = x) does not work in such a case.
In such a situation, we need to calculate the probability of X resting in an interval (a, b) along with P(a< X< b) which can take place using a PDF.
The Probability distribution function formula is defined as, F(x)= P(a < x < b)= ∫ba f(x)dx>0
Some instances where the Probability distribution function can work are:
- Temperature, rainfall and overall weather
- Time the computer takes to process input and give output
And many more.
Various applications of the probability density function (PDF) are:
- The PDF is used in shaping the data of atmospheric NOx temporal concentration yearly.
- It is treated to shape the diesel engine combustion.
- It works on the probabilities attached to random variables in statistics.

What is PMF?
The Probability Mass function depends on the values of any real number. It does not go to the value of X, which equals zero; in the case of x, the value of PMF is positive.
The PMF plays an important role in defining a discrete probability distribution and produces distinct outcomes. The formula of PMF is p(x)= P(X=x) i.e the probability of (x)= the probability (X=one specific x)
As it gives distinct values, PMF is very useful in computer programming and the shaping of statistics.
In simpler terms, probability mass function or PMS is a function that is associated with discrete events, i.e. probabilities related to those events occurring.
The word “mass“ explains the probabilities focused on discrete events.
Some of the applications of the probability mass function (PMF) are:
- The probability mass function (PMF) is central in statistics as it helps define the probabilities for discrete random variables.
- PMF is used to find the mean and variance of the distinct grouping.
- PMF is used in binomial and Poisson distributions where discrete values are used.
Some instances where the Probability mass function can work are:
- Number of students in a class
- Numbers on a dice
- Sides of a coin
- And many more.
Main Differences Between PDF and PMF
- The complete form of PDF is Probability Density Function, whereas the full form of PMF is Probability Mass Function.
- PMF is used when there is a need to find a solution in a range of discrete random variables, whereas PDF is used when there is a need to find a solution in a range of continuous random variables.
- PDF uses continuous random variables, whereas PMF uses discrete random variables.
- Pdf formula is F(x)= P(a < x < b)= ∫ba f(x)dx>0 whereas pmf formula is p(x)= P(X=x)
- The solutions of PDF fall in the radius of continuous random variables, whereas the solutions of PMF fall in the radius between numbers of discrete random variables
- https://amstat.tandfonline.com/doi/abs/10.1080/10485250701733747
- https://www.mitpressjournals.org/doi/abs/10.1162/0899766053723078
Last Updated : 11 June, 2023

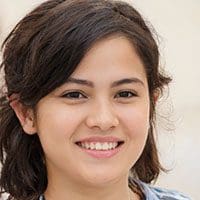
Emma Smith holds an MA degree in English from Irvine Valley College. She has been a Journalist since 2002, writing articles on the English language, Sports, and Law. Read more about me on her bio page.
This article provides a clear and detailed explanation of the difference between PDF and PMF. It’s very informative and helpful for anyone trying to understand these concepts.
I completely agree! The examples provided also make it easier to grasp the concepts.
The practical applications of PDF and PMF presented in this article make it a truly enlightening read. The examples used are very insightful.
Absolutely, the real-world applications add a lot of value to this article.
Agreed! It’s helpful to see how PDF and PMF are used in real-world scenarios.
The information about PDF and PMF is presented in a very organized and systematic manner. It’s easy to follow and comprehend.
Absolutely, the comparison table makes it even simpler to understand the distinctions between PDF and PMF.
The detailed explanations of the probability density function and probability mass function are very thorough and insightful. Great article!
I couldn’t agree more! This article is a valuable resource for understanding these statistical concepts.
The comparison table is a really effective way to illustrate the differences between PDF and PMF. It’s a commendable piece of writing.
Definitely! This article is a valuable resource for anyone looking to understand the nuances of PDF and PMF.
I couldn’t agree more. The clarity and conciseness of the comparison table make it easy to understand the distinctions between PDF and PMF.
I appreciate the way the article breaks down the applications of PDF and PMF in various fields. It shows the practical relevance of these concepts.
Definitely! It helps to see real-world examples of where PDF and PMF are used.
The information provided in this article about PDF and PMF is invaluable. It’s evident that a lot of research and expertise has gone into creating this content.
Absolutely, this article is a testament to the authors’ knowledge and ability to convey complex concepts in a clear and accessible way.
The authors of this article have done a fantastic job of providing a comprehensive understanding of PDF and PMF. It’s well-researched and clearly explained.
I agree, the depth of analysis and examples used make this article a standout in explaining PDF and PMF.
The article effectively communicates the key differences between PDF and PMF. It’s a great resource for students and professionals alike.
Absolutely, this is a very informative and well-written piece on PDF and PMF.
The explanations of PDF and PMF are presented in a very engaging and compelling manner. It’s a great read for anyone interested in statistics.
Absolutely! This article is a must-read for anyone wanting to grasp the concepts of PDF and PMF.
I completely agree. The article provides a comprehensive understanding of PDF and PMF in an accessible way.